Accelerating Data Analytics in the Cloud: David Krakov
Lee Razo talks to David Krakov, co-founder and CTO of Varada, an innovator in data virtualization, about how Varada accelerates large-scale data analytics to meet the challenge of rapidly growing data complexity.
Full Transcript
Welcome, David Krakov from Varada. A very - it's a great opportunity to speak with you. I've been learning all about Varada's technologies.
Varada is a platform or it creates a platform that enables data, scientist data, architects to accelerate and optimise workloads with adaptive indexing which is something I think we can go into a little bit later.
I was looking a little bit into your background. You have a pretty long and strong background in storage software development. And I noticed it's mixed up with a lot of leadership as well so it looks like you've been in all the different areas of the tech business.
Tell me a little bit about your background and how you came to identify this issue that led to founding Varada and what Varada is there to solve.
Sure. I'm very happy to be here speaking with you. So, as I said my background is software and storage. And like this company, I've been with this together with my co-founders, this was called XtremeIO in the space of all class storage, basically a company that's revolutionised the storage market and became the market leader in just three or four years.
And one of the things that made it possible for them is that they used hardware and software in different way. They identify what changed in the data centre, what changed in hardware, what changed and what is possible to do in the role, that software can exploit that or the software can leverage that.
And as a storage company of the business, increasingly, we're in the area of big data specifically enabling a better work as an Oracle as such.
And what we've seen, my co-founders and I as we launch Varada is that that kind of a revolution that the hardware enables the software to do differently has not been existent in the big data world who took a very specific course of how to handle big data that was mostly standing from the roots of the different approach to technology whether it's from Yahoo building at ADUF or from the data warehouse in [inaudible 00:04:03].
So, there's that on how you approach the problem technical wise.
And then on the other side, there's so much huge thing in that space.
And probably the last, the first six months or so of Varada we just spent talking to customers and learning even more and more about that thing and just, few different parts of that.
Even though they say that 80% of big data projects are doomed to fail.
This is like statistic that people would like to throw around and probably some part of that is just because companies when they start the big data project don't really know where they want to go.
But a lot of that comes to the actual problems of how you deal with that.
Some of it how do you even make sense of so much data whether it's from the collecting or even discovering it, knowing what you have.
And some of them being able to use that, to leverage that, to enable analyst or tools for the day to day where if you analyst, you got this idea, you got this insight.
You got something you need to do.
How fast can you get to position that? How fast can you get to create, to look into data, to find the right data, to get the right answer and to validating that.
And this is, and whilst we've seen this happening based on used cases or intel things so their enterprises.
They have the analyst theme.
They have this BI theme, but they also have seen that even more with companies who actually build data products.
Companies who built self-facing or customer facing, sometimes very large companies are still but products who are actually using data not to have this long- term data driven decision on the enterprise level but to actually do business from data.
It enables a security analyst to understand incidents in the network to enable marketing people to better understand the journeys of users across their sites.
And so, of course, this kind of - actually, data driven business and technology just really exposed all the deficiencies of the technology underneath. Because when you have this state of many, many, hundreds of thousands of people using this system at the same time, everybody expects an interactive answer.
Everybody expects to be able to work with the data, see graphs, see plots and expect that to happen consistently.
And there is no way for, take the engineering side, people who build that, there is no way for them to really be able to predict how people are going to use it.
It's just too wide.
Too wide, too large, too diverse, that is - so once you have those kinds of data products couple that with the whole, from data from suggested volume and velocity, this is extremely painful problem.
So, I will start we need to combine these two.
We need to be able to tackle this basic thing across being able to get facts from data without being able to predict specifically or model for a specific question.
And we need based on our old flash backgrounds that we can build software differently to leverage things that happen because you've never seen anyone in the tech side on the big data infrastructure doing that from first principles.
Yeah. And it's a good way to start because you're really describing the end state that we're at now.
We've been so successful with early with IT and with data that people, we take it for granted. It's like when I open up the tap into the sink, I expect water to come out. And I don't think about what it takes to actually get that water there from the source, right? And given your background in storage which I have as well, so I relate very much on the storage side of things. There's been a real history to get to this point, right.
That first the data started to become easier to collect so it grew.
We went from gigabytes to terabytes to petabytes and now exabytes.
And then the data sources then start to become more diverse, right? Their cameras, their servers, their people, whatever, all kinds of things that data comes from.
And so, then it starts to become more complex. So, now I guess then the infrastructure is now becoming complex, right? Now, we have Amazon and Ajer and we have object storage and whatever in this so I guess now we're seeing like a sheer complexity of data, just the data, itself, right? You've mentioned, we were talking earlier about the thousands of dimensions that someone has to deal with.
How do you approach that? How are people doing things before and how does Varada actually change how you deal with that kind of complexity?
Sure. So, all the things that mentioned maybe just what we exactly mean by that but the sort of so yeah, it does explain there is so much data but when you take the nuanced side of it, what's happening that makes it even harder.
There are two trends that happened. One is that the data itself goes to things like [inaudible 00:09:57] or object storage goes to, not to a system like we have in our house which is built for analytics.
It goes to storage.
And there's so much data coming from so many different sources and different life cycles, different phases so it's impossible to deal with how you consume it at the time you capture it.
It's easier just to throw it somewhere.
On the other hand, you got a lot of different kinds of data that people leverage daily.
Whether it's social data or sentiment behavioural data, IT data.
We all get tracked all the time by the Big Brother and every piece of data is collected.
And companies know how to leverage and use these sources of data to create more business.
So, it's not just about the size.
It's about the complexity.
Dimensions are just columns or tables.
But you've got so many different properties that you collect like a single click on the webpage can create 50 or 100 different properties to collect on that.
And just that click, you want to combine that from your data or from more data from other third-party sources.
So, and I'm a computer scientist from training so if you think like from the computer science perspective, when you double the data, it's a linear problem, how to double, what to do with that.
But if you double it's width, it's an exponential problem.
It's a much harder problem.
Because now you've got every possible combination and it's by power.
So, this makes it much harder.
And the second trend here, actually this is the trend on data but on the other side you said some things are like easy on like [inaudible 00:11:59] but that's part of what Cloud has been doing because then the older days when we say you got your Teradata, Oracle, you've got your organisational data warehouse and it's a large wreck and it is a provision for the year and the budget is very controlled by the IT and the size of it and you expand it and go and wait next year.
And so, the business units have to work within that boundary.
They're entirely dependent on IT to be able to allocate a piece of that and pie it among themselves on what to get. But the Cloud completely changed that game.
Part of the things that - it was essential the Cloud, having the Cloud that you can be, you can have essentially infinite resources at least for a point in time. You can have infinite storage on a Cloud [inaudible 00:13:01] go and analyse that.
And the power no longer sits with the IT with provisions to your wreck, it sits with users who can use just as much as they can which is a problem not only how do you deal with all the data but how do you also do that in a financial efficient way because the Cloud gave you the magic trick which is you can have infinite amount of resources if you just spend money on a computer problem and don't think about what are the hard problems that comes from boundaries and it will work but it will be very expensive. And more than expensive, it [inaudible 00:13:48] unpredictably expensive.
Yeah. (Laughter)
Because they might not be expensive, but they might be expensive long term.
So, now we're in a world and this world had come to Varada that's when we have to deal with that complexity of data. And to deal with that not in a brute force way because there is solution under that which is just [inaudible 00:14:21], if you're Facebook, if you're Uber, if you're Google, that probably works for you.
But now, how do you go efficiently about it?
Yeah. That's probably one of the advantages of the hyper scalers.
I think like you're saying I think that what has happened up until now is the way of dealing with this sort of problem, this complexity is to throw money at it basically just buy more computer power, but bigger and more you know.
When video came and helped the bit and then that also with the parallel processing within it also becomes another force money problem where you just throw more GPUs at it. And that so hyper scalers can do that but an ordinary business can't. They become dependent on that or they become limited in what they can do with their data, right? So, I guess it comes down to that old saying, you know work smarter not harder.
And right now, the solution is to work harder.
So, if you have that resource, you can solve the problem.
If you don't, you cannot.
So, that's a big problem so Varada has then found a way or at least put to use a way because probably the concept existed before but it needed to be applied to something called adaptive indexing whereas I understand that you even choose what kind of index to use depending on how the data is structured.
Is that right?
Yeah, sure.
So, just to give you where we are.
So, Varada is in the middle here so it's between data and data consumers.
Basically on the Cloud and mostly on the [inaudible 00:15:54] because this is where most of the complex are large and diverse big data comps do at least on cloud environments.
And as that middle tier, our job is give you fast performance, give that efficiently and give that for workloads that are hard to model in advance.
Because if you could, you might just build, data just on that.
It is like the known and unknown, and unknown, the unknown's problems, right? If you know already in advance what you don't know, you can structure it.
If not, then then you have to throw money and resource at it.
Yeah, sure.
So, I prefer the word predefined and not predefined because you know at the end, but you can't, the problem is too complex to define that in an efficient way.
So, these are the type of problems we have, we tackle.
The only alternative here is either going to model everything which is not possible when you get those kinds of workloads or we put it away.
So, the technology that we do combines two very strong and different forces into one that gives that ability.
They are adaptive in this thing which we'll talk about as you ask [inaudible 00:17:21].
So [inaudible 00:17:23] with the thing. There is, historically, there are [inaudible 00:17:32] using data for quite a bit of time but once we've moved in the world of data, you don't see the traditional types of indexes appear.
There are many technology reasons to that, most of them related to the fact that data can be stored in a cooler way so it's how you store that data to be efficient to process it.
And the fact that the way people can index the data today is just by sorting it and something and duplicating everything which is again not really possible when you have so much data [inaudible 00:18:15] duplicate that a few times but just different types of queries.
So, it's a very hard problem to solve because you got to deal with uncertainty of what the data is.
You have to deal with uncertainty of how it grows.
And you have to deal with the fact that there is so much of it that we're getting a piece of data, you can't really update anything behind.
You can't do any [inaudible 00:18:39] any vacuuming.
You have to be - keep your pace.
So, our adaptive index technology does exactly that.
So, let's just consider a piece of operational data.
I mean it doesn't really matter how many rows we have or how many columns we have as a goal to be met. So, what we do is we have this layer that's based on SSD's, it's cash flow indexes which leverages how SSD's work to deliver that.
We break the data into very small parts.
We call them analogue but essentially it maybe two kilobytes of a piece of a column.
And this might be a very small piece, say 60,000 piece of a column.
And then that very small piece of data and think about if we have kind of terabyte operational data, millions in that business.
From a small piece of data like that, we build an index just for it.
Now, that index the way it's built and how it does really depends on the contents of the data in just that piece.
If you have, like you have this column that [inaudible 00:20:02] sensor and it's between 70 and 72 then you got an index product for that for a numeric range of two degrees. You got user ID which is high technicity, a GUID.
You got an index for that.
You got text data.
You got an index to that.
And as your data changes because it does, within the data and its contents and distribution and why people ask of it which is [inaudible 00:20:36] analysis.
But as data changes, just imagine that loss.
So, you get the, we think about it as a match of millions of small indexes. Each one of them is optimal for a small part for the data.
It's entirely adaptive because each one of them is unique.
It's entirely dynamic because each one is independent of the others.
So, if data changes, and data comes in, data gets delivered, it has very small impact so just building a nano block.
And now every query becomes a walk or run across those small indexes which is also a kind of a [inaudible 00:21:24] is a very different level.
I can, we can instead of having run across a hundred terabytes of data, we can have rather than million indexes which shows small SSD pages and do that at a hundredth of a time for this inquiry and do that without knowing in advance where it's going to take us.
What accounts do I care about? What do I do with it? Because this fully covers and again, it's adapted to how data looks like and dynamic to how it changes.
So, this is a very big promise.
Yeah, so basically breaking the problem down into smaller problems and dealing with that and I guess there's a few advantages there of course that each of the smaller bit is more manageable.
Plus, you can do a lot in parallel and you're going to do them independently of each other, right? So, rather than taking and swallow the entire or what they say eat the whole elephant.
You just start with a bite. (Laughter) That makes a lot of sense.
And basically, turning big data into lots of small data and going from that point on.
So, that's a good overview on the technical side.
Switching over now to the mind of the business owner.
You know, the CEO, the product owner, the people who will be financially responsible for the projects being built on data.
Like I said now, data is taken for granted but everybody is trying to turn data into value and yet finding that when they open up that faucet, the water is not coming out as fast as they expected.
How should a business leader like a CEO look at this problem? What they should look at to determine whether this is an approach that they should take?
So, you can get this amount of business level, the actual fact on how you solve the problem is less important than the fact that there is a solution, more about it.
I think that from the organisational perspective, there are two types of value here.
One is just being able to do more with the data.
The performance, the performance worth the time to translate it to how much data you can access, is translated to how much the users, how sophisticated the features it can build if we made the product so that comes to the business value on what we can do with the data.
And the second comes to how much I can get an input. How fast can you read? And it's not only about cost because it's not only about the cost of having a very familiar and expert team, it's sometimes about the cost of not being on time and being able to deliver in a timely manner and respond fast to changes in what people need from data, how people use the data.
Because we go through the various words, relatively hard to predict where the nature of the problem due the nature of data being fast or the way to be fast, being fast in the way we handle the rest of the data is actually a business need by itself.
Basically, time to market is itself a business value and especially if your product is built from data then the faster you get there, the faster you get to market, more data that you can access, the higher quality your product becomes, right? So, it becomes a quality versus time to market type of trade off.
Yes.
It's, obviously cost has because technically cost is a part of it but cost looks like and it's expressed in the economics.
If you're building the product, you start so and so and [inaudible 00:25:28] customers so you have some time and economics you need to adhere to and that gives you some boundary of what you can do.
So, being efficient obviously expands what you can do.
But then it's about, what kind of question can you ask.
If the questions, if the query is in a second and not in 60 seconds, it's just some different type of analyst flow we can support with that and how fast it can get there. So, from a busines perspective, it's fast like different meanings of that.
That makes sense.
And then of course, there are obvious cases like you know in regards to data, we've heard about things like autonomous vehicles or algorithmic trading.
What are some common news cases that you're seeing that you're working with?
Sure. So, the types on these cases that we traditionally deal with today comes from areas of things like marketing analytics or clicks analytics for digital footprint.
We deal with, we have these cases of cybersecurity event analysis and collection and instant analysis.
We have some which is very similar with different space, root cause analysis and also, again from different space so we take a look at how the data looks likes.
You still have similarities in the areas of economics and medical research.
And some of the things you mentioned probably might be fit as well.
The thing about these kind of workloads is that they usually represent companies who are well matured on their journey to data but what's happened with data that's basically, every company that does not accelerate that, that does not have that at it's core is going to get left behind.
So, you know in the industry, new industry like cybersecurity, it's obviously that you can deal with data but then you get traditional industries like car manufacturing and those who are there and can be [inaudible 00:27:48] planning the journey.
So, there's a lot of different cases that fit year.
And I think that from the enterprise perspective, it's crucial to be able to know and understand that data can drive business.
And know and understand how to build an architecture on the technology side to be able to do that, to know the database later of building things that are going to limit you in a year or two.
And then....
I guess it's also something to think about, the decisions you take at the beginning of a path.
You won't feel the consequences until like further down the road, right? And then decisions you make when you first start building your infrastructure for collecting data and I think this is how a lot of companies end up having difficulties later is that you build the infrastructure for the data you have today and then it starts to scale and the infrastructure you built wasn't appropriate for that scale so then you end up later on with these problems that you didn't see coming until you hit that scale, right? So, one of the things that I've seen a lot of companies especially around data is they'll tell you, "Look, we can come with a solution. We can offer you numbers and benchmarks and everything but you don't really know what you have until you try it with your data.
It's unique also." How does Varada approach that as well? How do you engage a customer to see what their data situation is like?
Sure. Like one thing I think that companies just think about how the architecture looks like which is very important, it's from my perspective to keep in mind, it's thinking about the decoupling between how you get the data and how you consume that.
One of the hardest things to deal with later is tying in those two as, I think the data in and the way you're going to query that, inquiring the data in the way that was written.
And there are different technologies here, Datalex and others who can do all this kind of decoupling but I think at least from how does architecture should look today, this is a very big part of not running into a [inaudible 00:30:23] later.
And kind of decoupling enables for the same data, different users. Whether it's interactive analytics or data products with the product like ours or maybe [inaudible 00:30:38] or understanding that the quality and all of those different users for the same data or around the same data need that decoupling.
And for understanding so part of people asking question and saying what people can do with the data is a big problem.
And one of the things that we do as a product but we also offer that as a way for customers to just understand what's happening, understand that we can benefit or not or understand the properties of the workplace is work analysis.
The same way that it's so hard to predict and build model for something when you don't know...your workload is not defined enough.
It's hard for the data architects and data leaders to understand whether [inaudible 00:31:36].
You have the obvious stuff, you have a ticket about a query that keeps failing then yeah, that's fine without but that's just the tip of the iceberg.
And when you get thousands of queries a day, running across thousands of dimensions, it's really hard to know what's actually been used, what's actually important, where these experience deteriorates, where are the cost areas, because it was not built efficient enough so this kind of workflow analysis is covering the blind spot a lot of data things start to happen. And once the complexity grows so if we talk about products so we talk about indexing, the other side of that is workflow analysis because we can index everything but if you have few terabytes of storage, but we only use operationally a fraction of that indexing everything doesn't make that much sense.
But we can predict that. So, what we do is we have an analysis which looks at the queries, looks at how people use and have this [inaudible 00:32:56] behind to determine what's the operational asset.
Now, this comes from the product on site but externally, all of these understandings of how the workload looks like, what [inaudible 00:33:08], what changes, we share through very [inaudible 00:33:17] so you can go and understand what the workers do and reason about what's happening not on the specific query but the whole business workload and understanding how much it costs or does it deliver the right response they want, is it failing or not.
And this feasibility drives entirely how we decide what to optimise but gives a lot of value by itself.
And we figured out that and we started releasing some of those capabilities need open source route and we'll keep doing that because we figured out this is something that a lot of companies need as a way to understand what's happening.
We use that usually to show the value of the product by saying, hey, this workload can really benefit from that.
But I think that, with this, as we went through that open source route, it's nice that we get some, some customers won't use that.
Some customers [inaudible 00:34:22] because everybody wants to have a solution that can solve their problems and not use something that doesn't.
But then you as a customer, you have [inaudible 00:34:39] you can have just kind of workload analysis and understand more about it.
It's we can also understand a lot of patterns so that's one of the reasons why we started this open sourcing journey at that part.
Yeah. That's a big challenge, open sourcing as a business model but also, I mean the advantage you have effectively an unlimited development team in principle theoretically.
But on the other hand, a little bit less control over what comes out of it.
I've heard a lot of companies weighing that like what's the best way to go with open source.
What I like a lot though about the approach that you're taking is of course, one thing is to identify what's actually important, what is actually the business data that actually gets used but I think it also this approach of being able to I guess the word is ad hoc to think a little bit, be flexible about the types of questions you ask allows you to sort of learn and adapt as well.
You make a query.
You learn something.
You can change the query.
Whereas the traditional method, that's a lot more difficult because you have to determine everything in advance, right? And we see that benefit.
Yeah, sure.
And there's an assumption, you can know what's next and I mean.....
Yeah, exactly, yeah.
You don't know what you don't know. (Laughter)
Yeah. We did see so one of our design principles on that part of the work analysis engine that drives the indexing is that it's visible, it's explainable, it's controllable.
Because one thing we don't know from analysing queries is how important the workload is.
The business [inaudible 00:36:23] more or less and this is the knowledge that sits only with the domain of the customer.
And so, we use that visibility engine also the control to tell us what's important so to prioritise the indexing and this adds sort of business sense to, or the busines context to the data engineering work in a way that's relatively easy to reason about. Yeah, it can go and optimise the index and that way you can go in and control the bits of that.
And sometimes, you might want to do that but most of the time, the reason about it not specific query and the next one that comes but on a working level that has collection, different queries and you can hint sometimes the business more important than others.
And I think this is a powerful, when you think about it, it's new as a capability in terms of philosophy on how to go about this problem, how to deal with that unpredictability.
So, that's great.
I'm curious a little bit also on the leadership side of things.
Varada is of course a fast-growing company and a very, well, it's a hot space.
It's very competitive.
The tech business especially around data, there are certain technical areas, technological areas that are growing really fast.
What has it been like for you to lead a technical team through this? And how - do you find it challenging to keep your technical team connected to the business value of what you're providing? And how do you motivate your engineers to you know really know why they're waking up every day and what's the problem they're solving and why they do what they do.
Well, one of the reasons I work with a start-up, one of the nice things about a start-up at least if you build the company the right way is that everything is transparent.
And this whole team, everybody celebrates the wins.
Everybody feels the losses.
Whether it's about the bugs that the engineering had to deal with or the release or the other way around.
And by the way, very separate topic, one of the things that called our forces to work remote actually increased that kind of transparency.
As a team that's based in the US front facing, customer facing, sales and engineering in Israel, so with remote, how we build that but it still was an on site team here and there and moving to that more remote, increased that transparency and increased this culture of everybody knows what's happening. They're from the engineering from the site so....
That's amazing.
People got very used to working, or even people not in the tech business.
Like even your aunts and uncles now understand how to work remotely.
Yeah, I can Zoom with my grandma.
Yeah. (Laughter) Yeah, it's amazing.
So, yeah, indeed.
It's very hard to maintain like corporate politics in a small start-up.
I've worked in the past in a small company and I remember the VP of sales saying that if you work in this company, your first job is sales.
Your second job is whatever you were hired to do. (Laughter) So, it's always a really good one.
Where do you see Varada going in the next year?
I think we just - so the first, we just launched right about now, this months.
And we have so many doctors.
I think this is the year where we can move from this early phase where we know it works but we gather the maturity that we'd take to the market.
We're now at the point where we can onboard a lot of interesting [inaudible 00:40:36] cases.
We're not strictly very tech friendly on the [inaudible 00:40:44] enough to be able to integrate to be able to deliver by itself.
So, definitely our focus for this year is that.
While on this side, on the product side, it's not just about the indexing, the workload analysis gets smarter and more and more workloads we work on.
And one of the nice things building that way is that it [inaudible 00:41:15] not just for the growth of the company but for every customer that uses the product.
Because the more the engine gets smarter, the more everybody benefits.
So, I hope and I believe that we will see that kind of positive index cycle.
The more workloads, the work analysis engine learns and by the way, just to clear some worries that might come, the way the product works, it works within the customer environment.
So, no data ever leaves that premises of the customer, we're not the task solution.
We work with financial or the BAI and different industries with different concerns.
And it's fine because we don't actually- it doesn't leave the premises, the customer- except and only when there is a consent to entry.
So, teaching the engine how to work better, we don't really care about the query or data or anything like that.
And this is also with consent from the customers.
Yeah, that's (Overlapping Conversation) regulation and privacy and security.
These are big points when dealing with data.
And I would even, so yeah, that's an important point you make that data stays where it stays.
In some cases, like you know when it has to be encrypted or things like that but I would even say the other way around, this would allow you to know yourself what the data is and be more in compliance I think as a result.
Yeah. From a customer perspective obviously, all of this is about to be able to run something inside and use a task solution or external solution.
Once you have that kind, there's always types of data [inaudible 00:43:12] because relational because some contractual obligation of third parties.
And so whatever task solution you have, it becomes [inaudible 00:43:23].
Because not all data can move there.
They might have organisation or different life cycle so just being able to control everything within.
It doesn't matter which technology you use but it adds value by itself.
It makes the compliance and the security storage easier [inaudible 00:43:51] to do it.
Yeah, that's a big, big, big challenge there.
And also working with companies that do services in the cloud and first of all, they have to assure customers that they don't in any way introduce any risk but secondly, just going to the cloud is already a big challenge because of these issues so that's very important that you say that.
And speaking of that topic of cloud so obviously we're called Cloud Native X and Cloud Native is one of these words that have gone around the last years.
I'm sure you're familiar with it.
And I've always wondered when I first heard the word, I'm like, cloud native.
Well, that makes sense.
It probably means that it was something that was born in the cloud, right? And then later it came to me in something else.
The [inaudible 00:44:36] cloud native computing foundation gave it a definition.
What do you think it means? What does that word cloud native mean to you? How would you define it?
I think it's a manner of thinking.
It's a manner of you know I'm an immigrant, so from an another place.
I was born in the Soviet Union and moved to Israel when I was kid.
And every immigrant relates to that transformation of changing the language that you think in and the culture that you operate in, what you can do, what you can't do. Before I can start to think as a local.
And some people never go through that.
Some people struggle that for a long time.
And this kind of transformation so I'm taking it on personal level but Cloud is just so different in the way that you have to think about [inaudible 00:45:41].
You have to think about solutions. You have to think about - realise that it's about how you use these resources and realise how you [inaudible 00:45:52].
It's actually like completely changing the language that you're speaking and the culture that you work in.
So, I think that cloud native is about doing that transformation in the way of thinking.
There's one reason why hybrid technology still struggles to find the best practices there because it's just, one it's a bit getting to another so a small way to work like the other losing, it doesn't matter which way you do that first thing but [inaudible 00:46:30].
Yeah. I love that answer. And personally, I'm also an immigrated Mexican-American who lives in the Netherlands so I completely relate to everything you're saying. (Laughter) Moving from one place to another.
In a way, you never really become completely local but you can make an effort and get there and you can tell immediately that difference of someone who is actually local.
So, that's great answer.
I think obviously as time goes on, more and more things, I think the term will go away actually.
I think it will just become IT again.
(Laughter) So, that's really great.
I really appreciate it.
Is there anything else you would like to cover before we wrap up?
No.
I'm....
Perfect.
Okay.
I really appreciate your time.
Great chat.
I really love learning about, doing different technologies. I'd say a lot of the people I talk to solve problems that we only created a few years ago so all the step is really new and I'm glad that we could give a preview a little bit for people who are just getting introduced to this as I think this sort of solution is going to become more and more important so looking very much forward to working with you further and speaking with you again soon hopefully.
Thanks very much.
Thank you very much for your time. Thank you.
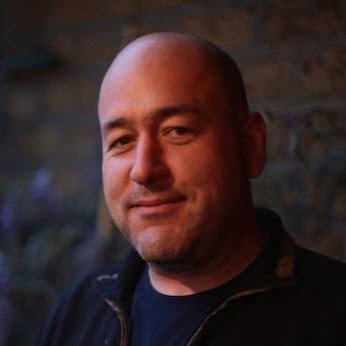
Lee Razo
Consultant, CloudNativeX
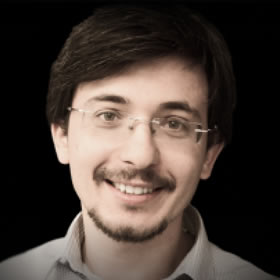